Robotics:Nothing To Everything
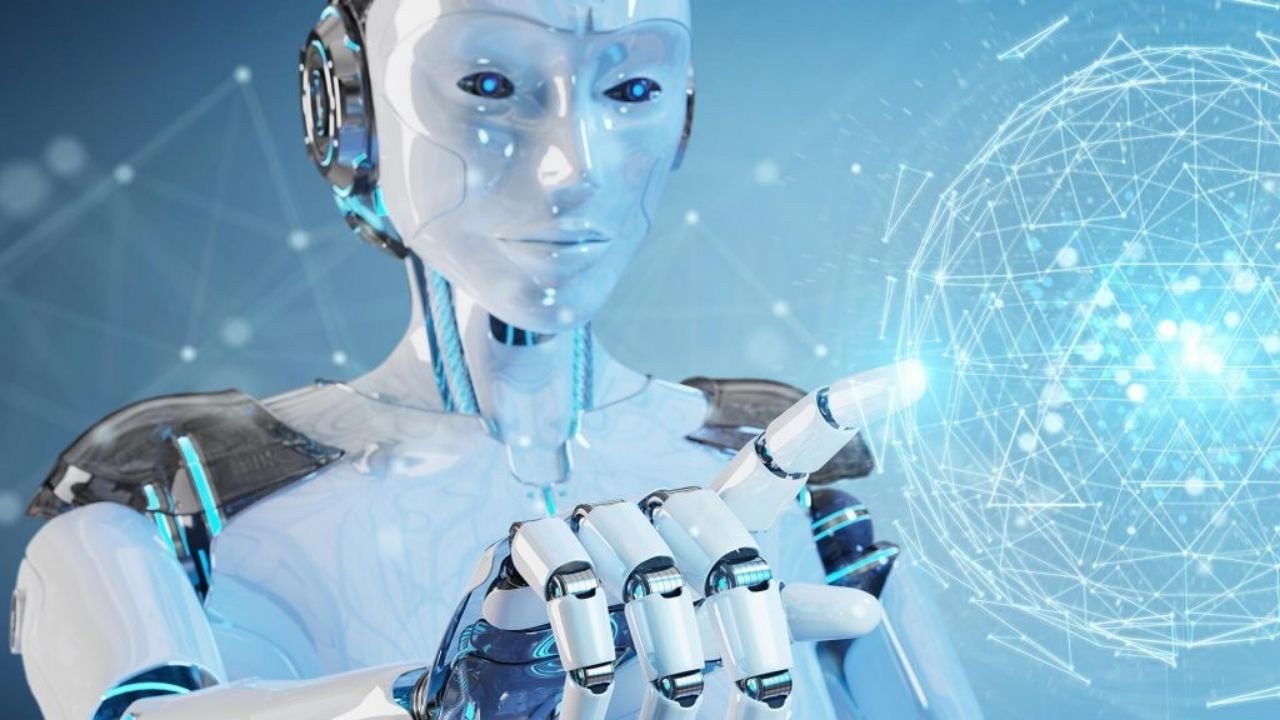
Imagine driving your bike up a number of stairs — hundreds of steps. If you are not a talented cyclist, you probably might fall down and hurt yourself. Wheels operate very well in a highly organized environment—on a flat surface and rare traffic crossing your travel path. In mechatronics, people would suggest that the environment for wheeled robots should be operated relatively with similar constraints. Under these constraints, the wheels are very effective and function smoothly and we have a supply chain of components for wheels, axles, and steering components.
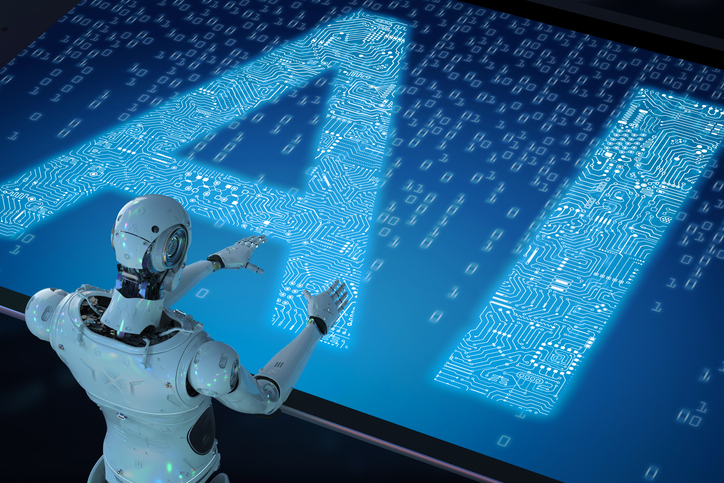
But the stairs are less regulated and dynamic environments. Stairs in a single building can have different heights. They can change direction, turn 180 degrees on themselves. They can also be straight, elliptical, or irregular. Stairs require more kinesthetic and spatial intelligence than flat surfaces. For mechatronic engineers, they face a host of challenges in the process of developing robots. Especially, for a world that goes beyond flat, defined surfaces.
Flat Country
In 1959, at General Motors in Trenton New Jersey, Joseph Engelberger installed his robotics arm Unimate #001 to capture diecast components. This system had no sensors. It had a memory of nearly 64K. Only in very managed and repetitive open-loop conditions can it be used safely. “Open Loop” refers to the concept that the system does not feel as “closing the loop” is sensing. The Unimate could perform only one task it was designed to use its 64K memory.
Compared to Unimate #001, in regulated environments, modern appliance mechatronics performs miracles. For example your laundry room. Modern washers are more meaningful than Unimate #001. They weigh the wash with stress gauges or measure the energy needed to shift the load. Dry people can feel how much moisture is evaporating as they dry, so they know when to stop.
New sensors and their processors interpret data to allow our daily device to close the circuit. These digital sensors and processors can be reliable, cost-effective. They are also precise because they take small data samples and use simple algorithms.
Space of high dimensions
We live in very diverse environments. Various stairs and room types, smooth floors and tapestry floors, wet and dry, noisy and quiet. For robotic machines to travel productively inside our world, they will need a four-legged animal’s kinestic intelligence. To put this in perspective, it is believed that the first vertebrate (hagfish) arrived 450 million years ago. The first four-legged vertebrate was tetrapod 390 million years ago. So the unstructured world of primordial earth took around 60 million years to navigate.
Boston Dynamics only developed a $74,000 spot called a four-legged robot that can repetitively follow a course in a known environment 62 years after the release of Unimate #001. Since it is mobile, it needs sufficient onboard processing to remember the route and the step instructions. In this paragon of modern technology, the kinesthetic intelligence (KI) of the tetrapod is not yet present. It can float on smooth surfaces, has difficulties navigating objects, and still struggles on steps.
How much doggy is there in the window?
US organizations, for 9.1 million people employed in production-based jobs, spend $300 billion a year on salaries — activities that include repeated heavy lifting, transporting machinery, and materials. The organizations spend 15 billion dollars on salaries for the completion of these tasks alone (BLS, 2016), for people who are very dexterous and working well together, their excitement is fuelled by new circumstances. For each of these individuals, the full strain on an organisation is about $40,000–$60,000 a year.
Spot, entirely burdened at more than $150,000 a year, can only perform the most repetitive tasks. And he is still at risk of being hit or hurt (see HitchBot). In order to be a valuable collaborator of a human employee, robots must either surpass their success in tight tasks, become much cheaper to own and work or can perform risky work that threatens people’s lives. Consider, for example, a robot-human team that telephones a robot in a dirty, dark or hazardous (3D) environment – like Sarcos Guardian S’s inspection of an asbestos plant.
Continued Smart Agent Evolution…
Organisms develop primarily because of circumstances, stimuli and the ability to adapt and prosper in a particular environment. In the case of robotics, environment needs, technological progress and the ability to build a device in a commercially viable manner are the main factors to evolutionary success.
The economy of hardware and mechatronic companies does not change with software or silicon. However, edge computational success takes costs down and parallel increases, thus deep learning leads to increased productivity and more capacities beyond the reach of business scenarios. Since the sensing of AI and machine learning (e.g. object recognition, detection of poses, identification of motion objects) are commodities they can lead to a reduced level of mechatronics interest. Perhaps they can also motivate mechatronic open source hardware platforms.
Successful technologies can operate at viable prices and performance points in retail, delivery, and security markets. The ability to link these robots into larger networks will allow us to close business loops and achieve digital transformation as Networking and Edge Computing evolve.
Every automation period in these markets exposes a cycle of changes from research projects to profitable businesses – and increasingly smart agents mark each epoch. I started coding when I was 14, but now more than ever I’m excited to see how technology makes our lives more productive, safer, and fulfilling.
Also Read: Training a Robot (Using Artificial Intelligence and Supercomputers) Is Just Amazing
The Entrepreneurs Diaries is now available on Telegram. Join our telegram channel to get instant updates from TED.